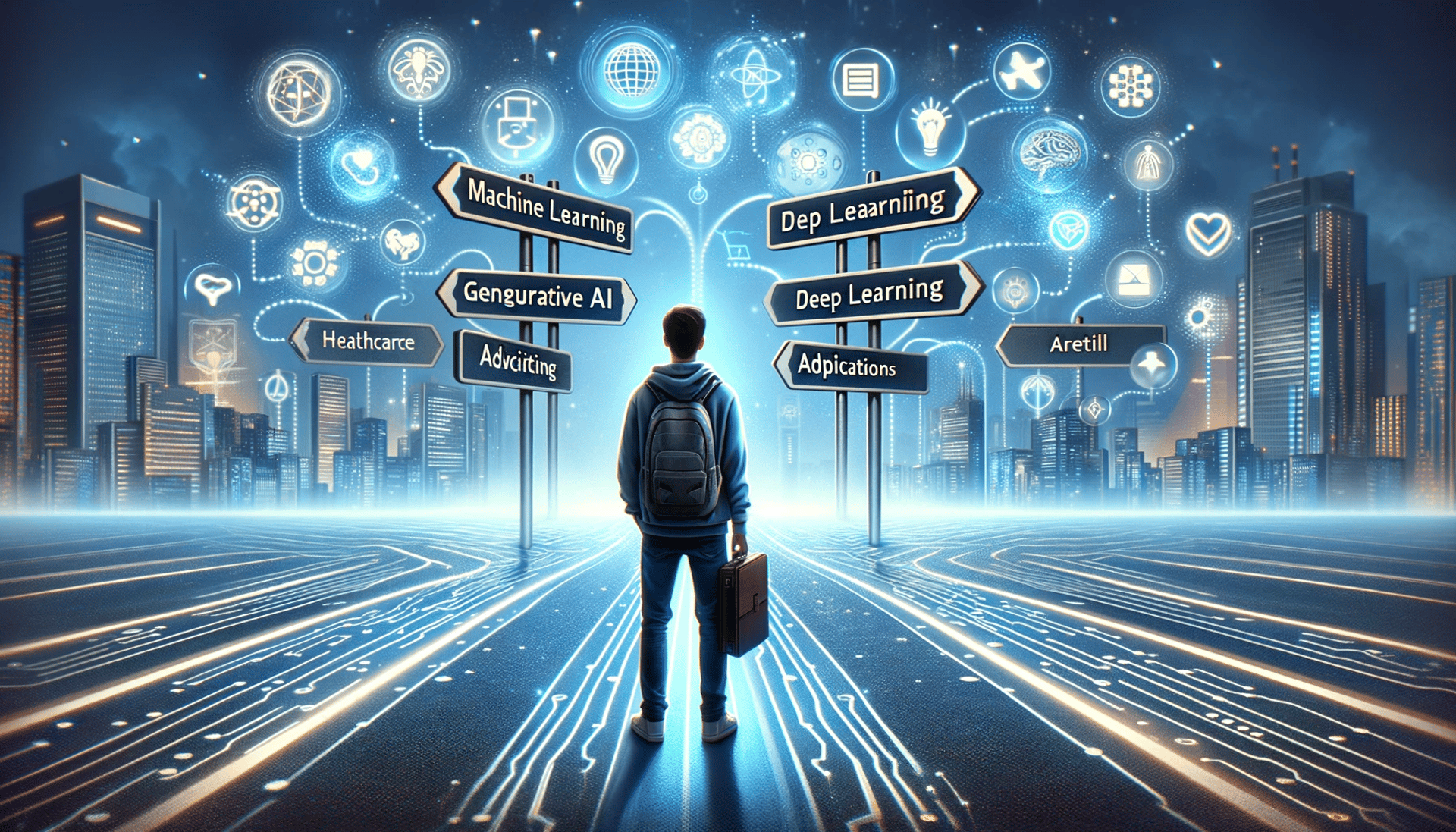
What advice would I give to a student (or any engineer!) eager to specialize in AI?
After enduring 40 years of fluctuating fortunes, often referred to as 'AI Winters,' AI began to significantly advance over the past 10-15 years. This was largely due to the widespread adoption of Machine Learning algorithms, notably (Deep) Neural Networks, which found applications in various fields including vision, image classification, voice, ... However, the real game-changer was the surge of innovation brought about by Generative AI in 2022. This led to an explosion of products and applications in numerous areas such as IT, Healthcare, Advertising, Industries, Legal and Retail among others. AI is one of these technologies that will not follow a smooth, untroubled path; there will be high promises and setbacks along the way but AI will definitely be there to stay.
So, what advice would I give to a student (or any engineer!) eager to specialize in AI?
Five years ago, I might have recommended studying fundamental sciences like mathematics and statistics, likely within the context of Data Science, as the importance of 'understanding' data began to really emerge. Three years ago, my advice would have been to concentrate on core ML algorithms such as Deep Learning, along with the tools involved in MLOps, but the world has changed (for the good) unexpectedly and quickly:
- Widespread adoption of ML/AI, particularly Gen-AI algorithms, across various industries, and this is just the beginning. It won't be long before every application is AI-enabled with an assistant or co-pilot capable of data analysis and, in the near future, more advanced planning and reasoning capabilities. In fact, many applications are already powered by AI and Gen-AI for text processing and other content generation such as voice and video.
- Development of AI/ML platforms offered as a service. A few years ago, simple data processing tasks required hours of a data scientist's time for collecting large datasets, performing data cleanup, and using tools like Pandas for data analysis or even training models for tasks like correlation analysis, trend identification, classification, regression, or forecasting. These tasks can now be performed with just a few clicks thanks to as-as-service data science and AI; this does not mean that one should have get a good understanding of the underlying technologies so as to correctly interpret and correct outcomes, especially when dealing with product development powered by AI.
- AI Open Innovation leading to the development of numerous codebases, models, and tools.
Does this mean that advanced ML/AI engineers are no longer necessary? Not at all! Such professionals will continue to be in high demand by many companies.
To conclude, here are some recommendations I would make to someone deeply interested in AI, especially a student beginning their university studies:
- Have a strong foundation in fundamental sciences: mathematics, statistics, and your chosen science field.
- In this fast-moving world of technological innovation, be a multi-disciplinary specialist: Healthcare & AI, Engineering & AI, IT & AI, etc. Don't ignore AI, or you risk being left behind. Become a proficient AI practitioner with a thorough understanding of the underlying technologies, even when they're not required due to the availability of tools. In my opinion, it's the combination of deep expertise in a specific field (like healthcare, advertising, networking, automotive) and a strong grasp of AI technologies that will make your profile unique and irreplaceable. AI alone will never replace subject matter expertise, and subject matter expertise will soon be no longer sufficient.